Exploring Image Datasets for Object Detection: A Comprehensive Guide
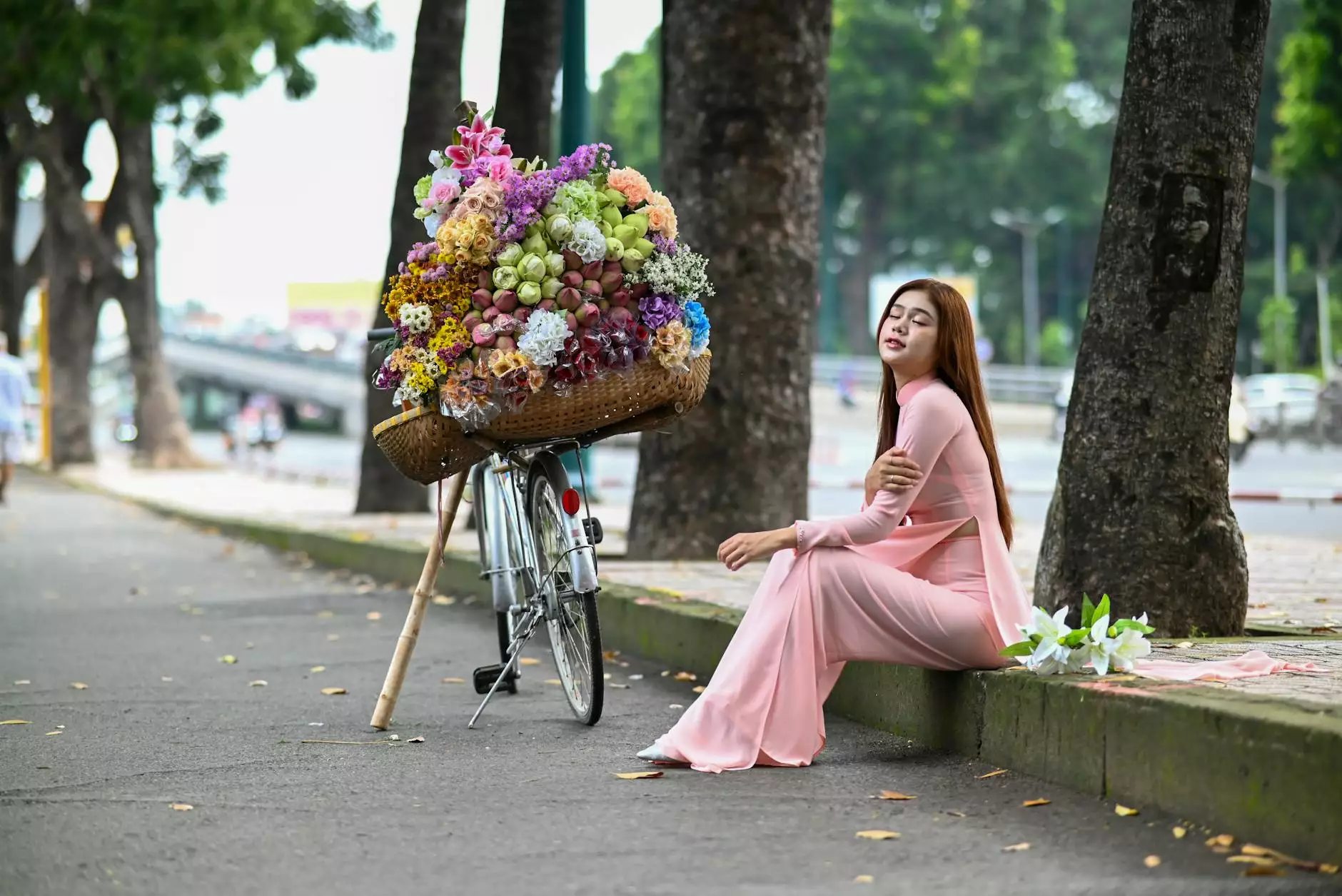
In the rapidly evolving field of artificial intelligence and machine learning, the importance of a robust image dataset for object detection cannot be overstated. As businesses increasingly leverage computer vision technologies, understanding the nuances of image datasets is vital not only for developers but for organizations aiming to incorporate cutting-edge solutions into their operations. At Keymakr.com, we recognize the transformative power of these datasets and are committed to delivering superior quality solutions for our clients.
Understanding Object Detection
Object detection is a crucial aspect of computer vision, enabling machines to identify and locate objects within images or videos. This technology is foundational in myriad applications, including:
- Autonomous Vehicles - Recognizing pedestrians, road signs, and other vehicles.
- Medical Imaging - Identifying anomalies in scans for better diagnosis.
- Retail and Inventory Management - Tracking products and monitoring stock levels.
- Surveillance Systems - Detecting unusual activity in monitored environments.
The Role of Image Datasets in Object Detection
Image datasets are collections of images used to train object detection models. They play a pivotal role by providing the necessary data for algorithms to learn patterns and features critical for accurately identifying and classifying objects. Here’s why a high-quality image dataset for object detection is essential:
1. Data Diversity
Diversity in data is paramount. A comprehensive dataset should encompass a broad range of conditions, including:
- Various lighting conditions
- Different angles and perspectives
- Multiple backgrounds and environments
- Varying sizes and shapes of objects
2. Annotated Data for Supervised Learning
For supervised learning approaches, annotated images are crucial. These annotations pinpoint the locations and classifications of objects within the images. An effective image dataset for object detection typically includes:
- Bounding Boxes - Rectangles around the objects in the images.
- Class Labels - Identifying what each object is.
- Segmentation Maps - Distinguishing the object from the background.
3. Large Dataset Size
A larger dataset enhances the model's ability to generalize from training data to unseen data. Small datasets may lead to overfitting, where the model performs well on training data but poorly on real-world applications. At Keymakr, we understand that obtaining a sufficiently large image dataset for object detection ensures better performance and reliability.
Types of Image Datasets Used in Object Detection
There are various types of image datasets utilized in the field of object detection. The choice of dataset can greatly influence the performance of the detection models. Here are some of the most notable ones:
1. Publicly Available Datasets
Numerous publicly available datasets allow developers to kickstart their projects without the need to create datasets from scratch. Some popular options include:
- COCO (Common Objects in Context) - Widely used for training deep learning models, featuring over 300,000 images with extensive annotations.
- PASCAL VOC - Contains various object categories, offering significant annotations for tasks such as detection and segmentation.
- Open Images - A vast dataset with bounding boxes and segmentations for a variety of object classes.
2. Custom Datasets
For specific applications and industries, creating a custom dataset may be necessary. This process involves:
- Collecting Images - Gathering images that reflect the environment and object types relevant to the business's application.
- Annotating Data - Utilizing professional annotators or automated tools to ensure accuracy in labeling.
- Data Augmentation - Applying techniques to increase dataset variability, such as flipping, cropping, and adding noise to images.
How Keymakr.com Enhances Your Image Dataset for Object Detection
At Keymakr.com, we specialize in refining your image datasets for optimal object detection performance. Here’s how we can help:
1. High-Quality Data Annotation
The precision of object detection greatly relies on the quality of annotations. Our team utilizes an extensive range of tools and methodologies to provide accurate annotations, including:
- Expert reviewers for complex annotation tasks.
- Automated tools to ensure consistency and speed.
2. Dataset Expansion
We recognize that a larger dataset enhances model performance. We utilize advanced data augmentation techniques to artificially increase dataset size without compromising quality. This ensures your models learn effectively from diverse data points.
3. Custom Dataset Solutions
Understanding that one size does not fit all, we offer tailored solutions that focus on your specific business needs. Our consultants work with you to identify essential object classes and gather relevant images, ensuring the dataset is a perfect fit for your application.
Challenges in Creating Quality Image Datasets
While the advantages of quality datasets are clear, the path to creating them can come with challenges:
1. Resource Intensive
Gathering and annotating images can be a time-consuming and costly endeavor, requiring skilled labor and significant time investment.
2. Ensuring Quality and Consistency
Variance in quality and style of annotations can lead to poor model performance. Establishing strict guidelines and using multiple annotators can help mitigate this issue.
3. Keeping Up with Changing Data
As environments change, so too should your datasets. Continuous updates are necessary to maintain relevance and accuracy in object detection tasks.
The Future of Image Datasets for Object Detection
As the field of AI continues to advance, the methodologies for creating and utilizing image datasets for object detection are also evolving. Some emerging trends include:
- Self-Supervised Learning - Techniques that reduce the reliance on labeled data by leveraging unlabeled data.
- Transfer Learning - Utilizing pre-trained models on large datasets to improve results on smaller, specific datasets.
- Collaborative Datasets - Sharing datasets among organizations to enhance diversity and utility in object detection models.
Conclusion
In conclusion, the significance of a high-quality image dataset for object detection is evident in its ability to revolutionize numerous sectors by enabling machines to understand and interpret visual data. With businesses like Keymakr.com at the forefront, organizations can navigate this complex landscape with expert guidance and tailor-fit solutions. By harnessing the power of image datasets, your business can stay ahead of the technological curve and implement innovative solutions that drive success.
To learn more about how we can help you develop your image dataset for object detection, visit Keymakr.com today!